In the digital twin paradigm, system diagnostics-informed system prognostics are used for optimization of system maintenance or operational tasks. System diagnostics are used to ascertain the current health or state of the system. This information, when integrated into a system prognostics framework, helps provide the most up-to-date estimate of system behavior. The updated system behavior model can be used for making decisions regarding maintenance scheduling or effective operations.
Digital twin to design damage-adaptive rotorcraft maneuvers
- Monitor and retard degradation of critical rotorcraft components
- Stress analysis models (ABAQUS), rotorcraft dynamics models (RCAS) and component strain data
- Minimize the critical load acting on the component
- Ensure safe completion of the mission and satisfaction of application-specific constraints (distance, altitude and/or time of flight)
Digital twin for intelligent maritime vessel maintenance and operations
- Monitoring and prognosis of the ship fatigue damage accumulation to support maintenance and operational decisions
- Fatigue damage inferred from the observed data and vessel-specific analysis models
- Digital Twin created by integrating the following data-driven and analysis models, as well as vessel structure and load data
- Vessel-specific maintenance and/or operational decisions can be made using the digital twin
Data: |
Analysis Models: |
|
|
Digital Twin in Additive Manufacturing
- Monitor and improve the quality of an additively manufactured part
- Integrate physics-based simulation models with online monitoring data acquired from advanced sensors during the manufacturing process
- Diagnosis of the quantity of interest (QoI) e.g. porosity/voids, deformation, geometry etc. in the partially manufactured part
- Adjust process parameters in future layers to manufacture parts of desired quality
Aircraft Wing Health Monitoring Digital Twin
- Track fatigue crack growth in an aircraft wing to inform maintenance decisions
- Use a dynamic Bayesian network to build a versatile probabilistic model for diagnosis and prognosis
- Integrate physics models and various aleatory and epistemic uncertainty sources in crack growth prediction
Funding
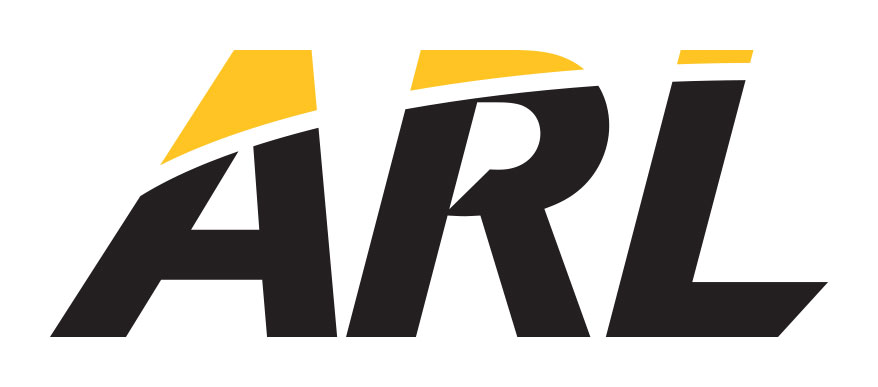
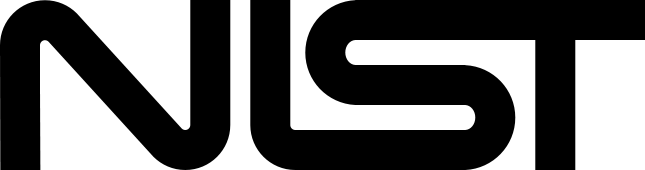
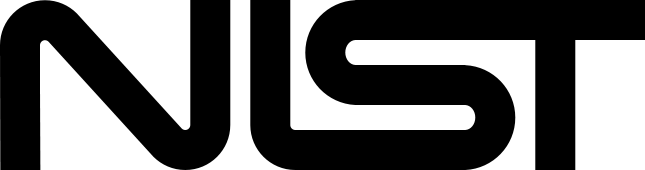
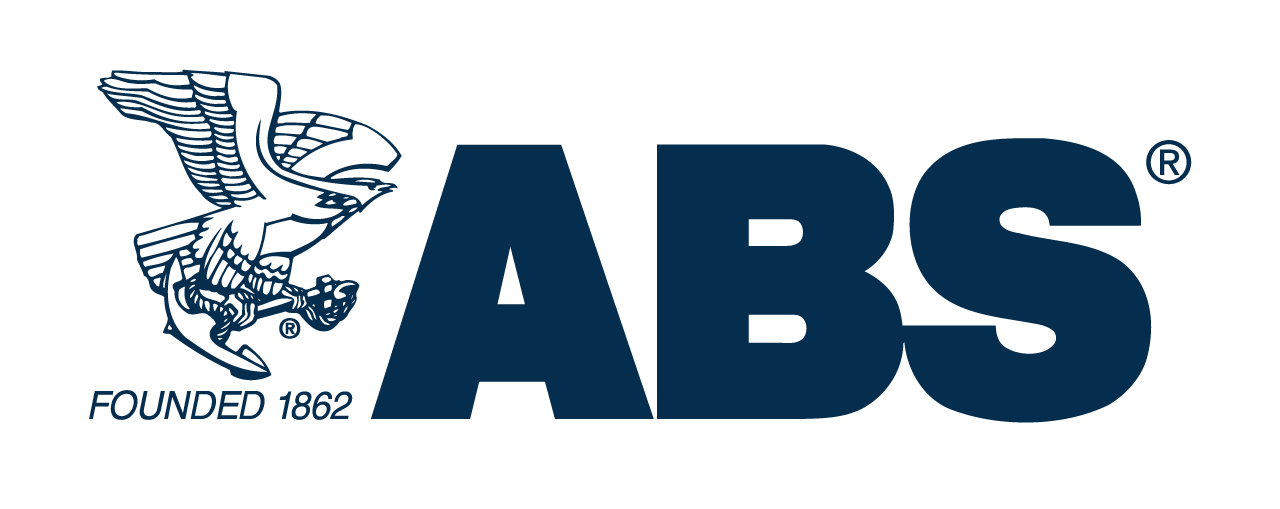
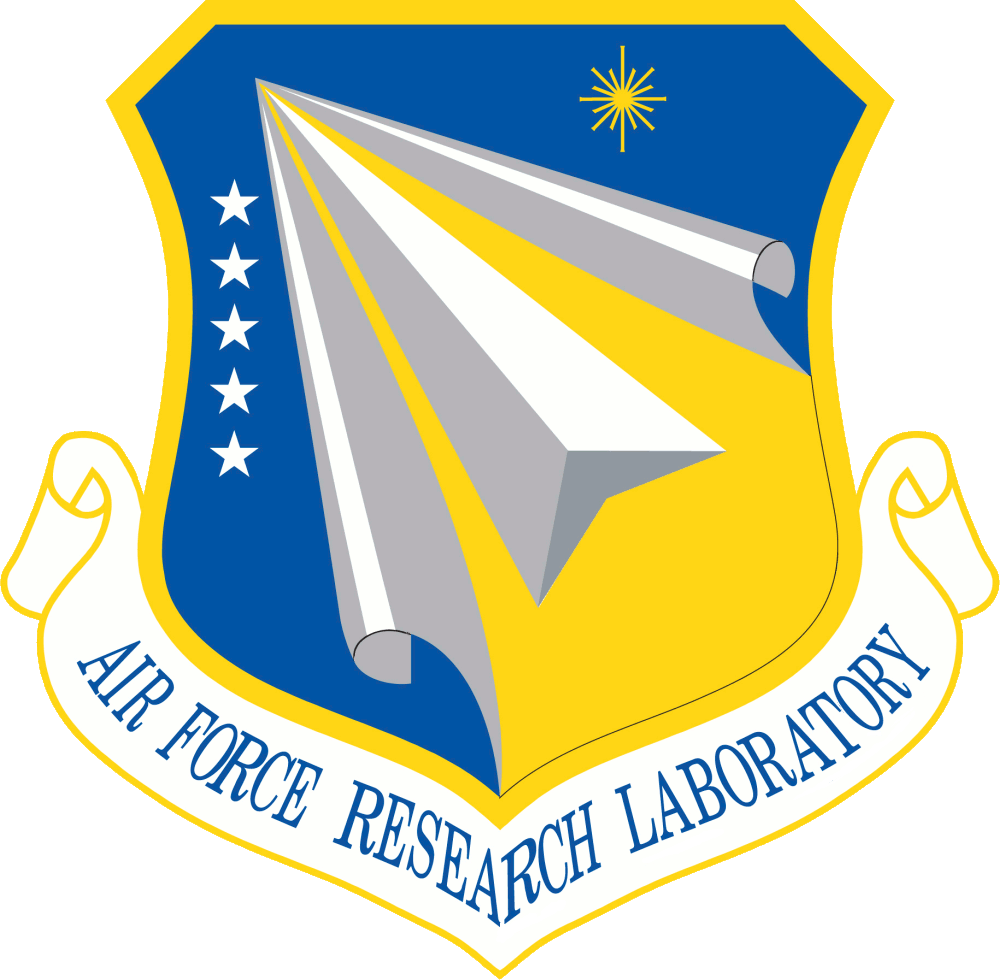
Current People
- Sankaran Mahadevan, Professor
- Pranav Karve, Research Assistant Professor
- Paromita Nath, Postdoctoral Research Scholar
- Eric Vanderhorn, Ph.D. Student
- Yulin Guo, Ph.D. Student
- Berkcan Kapusuzoglu, Ph.D. Student
- William Sisson, Ph.D. Student
Publications
2. Li, C., Mahadevan, S., Ling, Y., Choze, S., & Wang, L, “Dynamic Bayesian Network for Aircraft Wing Health Monitoring Digital Twin”. AIAA Journal, Vol. 55, No. 3, pp 930-941, 2016.
3. Nannapaneni, S., Mahadevan, S., Dubey, A., & Lee. Y. T. T., "Online monitoring and control of a cyber-physical manufacturing process under uncertainty," Journal of Intelligent Manufacturing, 2020.
4. VanDerHorn, E., & Mahadevan, S., "Digital Twin: Generalization, characterization and implementation," Decision Support Systems, 2021.
5. VanDerHorn, E., Wang, Z., & Mahadevan, S., "Towards a Digital Twin Approach for Vessel-Specific Fatigue Damage Monitoring and Prognosis". Reliability Engineering & System Safety, 2021.